On January 31, 2024, Tencent’s AI Lab unveiled YOLO-World, a groundbreaking model in real-time, open-vocabulary object detection. This zero-shot model eliminates the need for prior training, allowing for the detection of any object by simply defining a prompt.
Key Feature: Real-time object detection with just a prompt.
Accessibility: The model is accessible on the YOLO-World Github.
YOLO-World addresses the speed limitations of previous zero-shot object detection models, leveraging the CNN-based YOLO architecture for unmatched speed and accuracy.
Transitioning to YOLO-World
Traditional object detection models were limited by their training datasets, restricting their ability to identify objects outside predefined categories. Open-vocabulary object detection (OVD) models emerged to recognize objects beyond these categories using large-scale image-text data training. However, most relied on the slower Transformer architecture, introducing delays not suitable for real-time applications.
Introducing YOLO-World
YOLO-World represents a significant advancement, proving that lightweight models can offer robust performance in open-vocabulary object detection, crucial for applications demanding efficiency and speed.
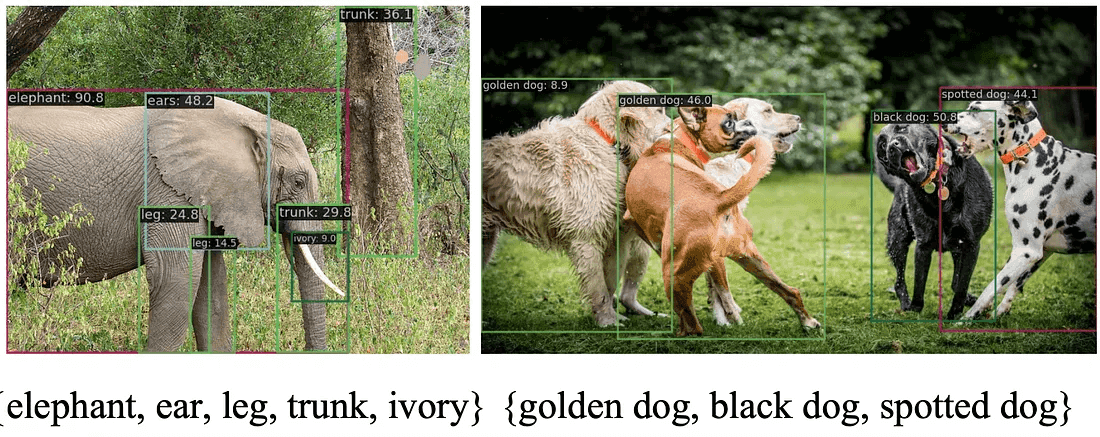
YOLO-world on sample images
Groundbreaking Features:
Prompt-then-Detect Paradigm: Avoids real-time text encoding, significantly reducing computational demand.
Dynamic Detection Vocabulary: Allows for the easy adjustment of the detection vocabulary to meet user needs.
YOLO-World Architecture
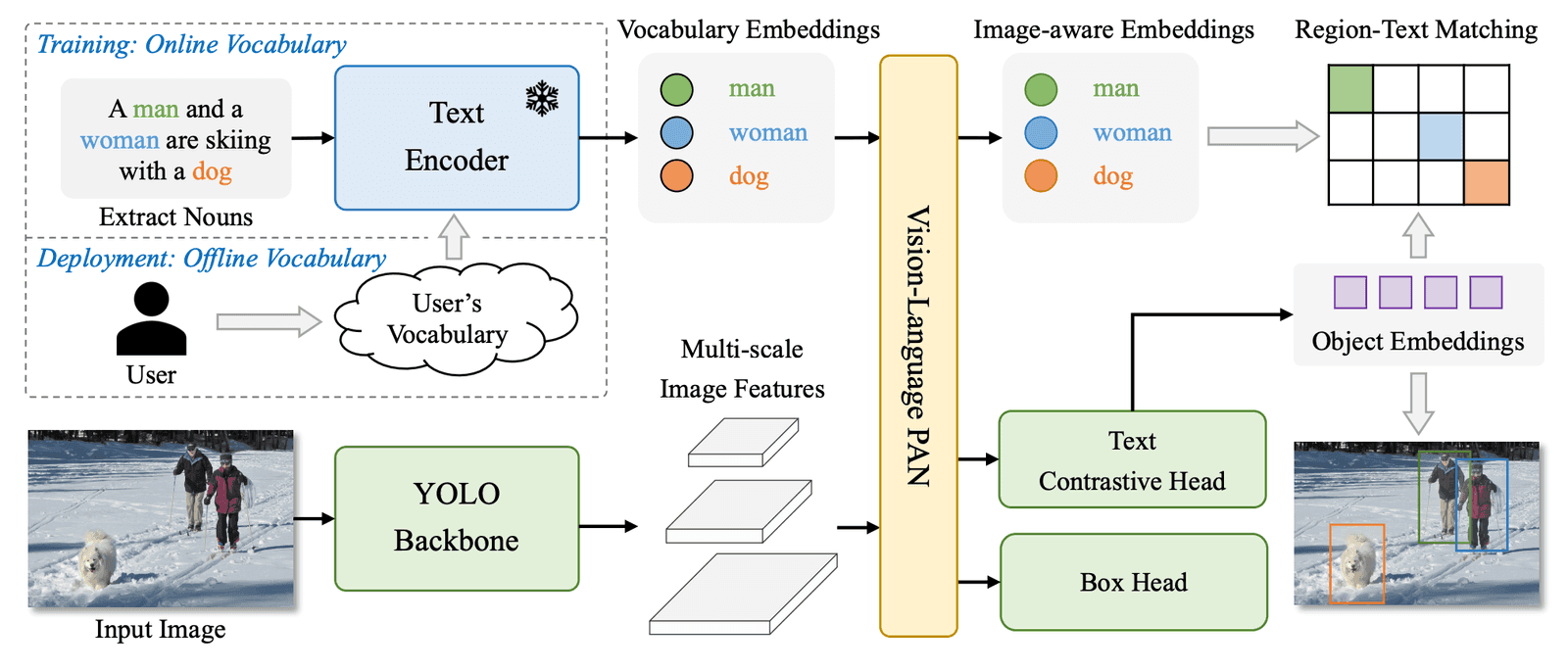
Image Description: Overall Architecture of YOLO-World. Source YOLO-World paper.
YOLO-World's architecture is designed to efficiently fuse image features with text embeddings, consisting of:
YOLO Detector: Extracts multi-scale features from images using Ultralytics YOLOv8.
Text Encoder: Uses a Transformer-based encoder pre-trained by OpenAI’s CLIP.
RepVL-PAN: Performs multi-level cross-modality fusion between image features and text embeddings.
The fusion process involves text-guided feature modulation and image-pooling attention, optimizing detection accuracy.
Performance and Usage
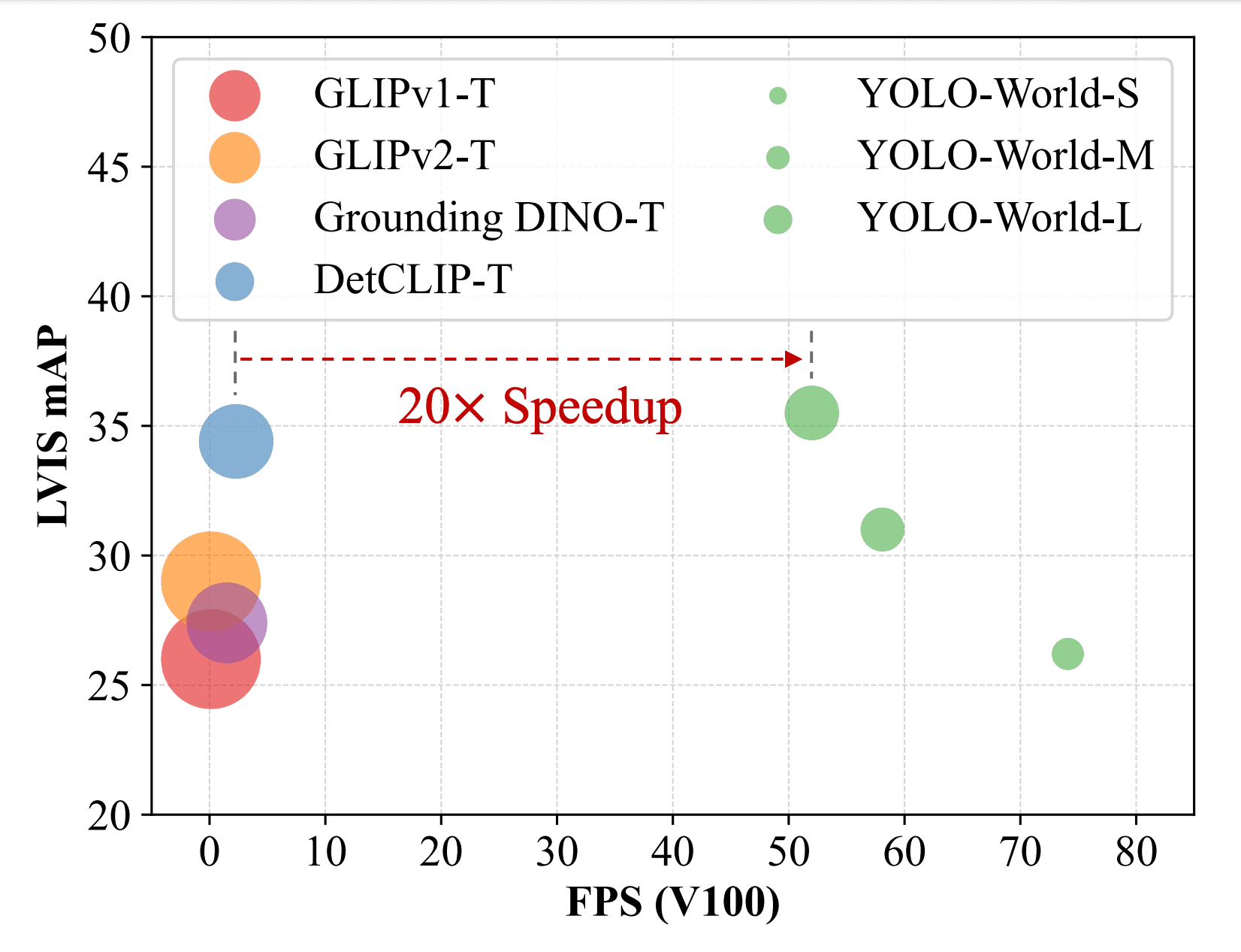
Comparison of YOLO-World with the latest open vocabulary methods in terms of speed and accuracy. Models were compared on the LVIS dataset and measured on NVIDIA V100. Source YOLO-World paper.
YOLO-World achieves impressive accuracy and speed on the LVIS dataset without needing specialized acceleration techniques. It's ideal for real-time object tracking, video analytics, and auto-labeling for model training, enabling the creation of vision applications without extensive data labeling and training.
Comprehensive Breakdown and Guide to YOLO-World
Overcoming Limitations of Previous Models
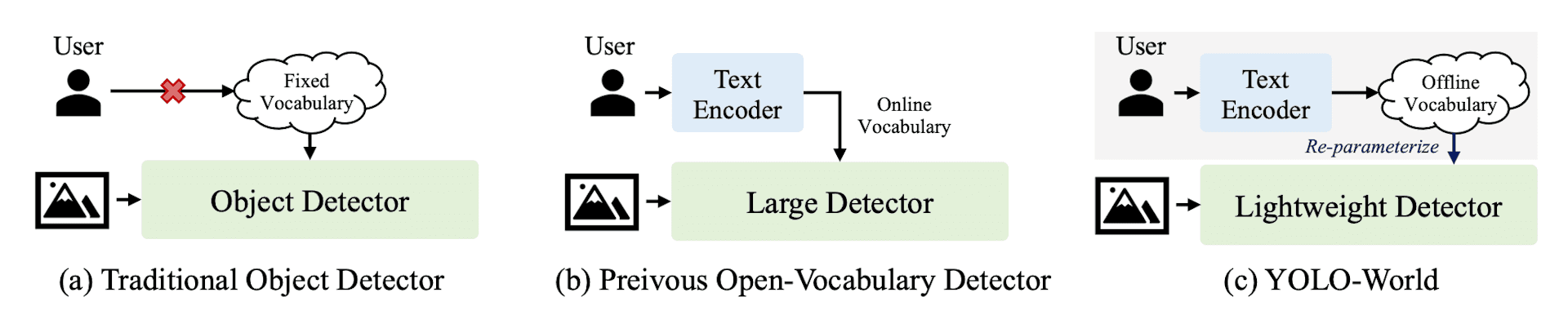
Comparison of different object detection inference paradigms. Source YOLO-World paper.
Models like Grounding DINO and OWL-ViT, despite their advancements in open-vocabulary object detection, were hindered by their reliance on the Transformer architecture. These models, while powerful, were not suitable for real-time applications due to their slower processing times. YOLO-World, leveraging the faster CNN-based YOLO architecture, addresses these limitations, offering real-time performance without sacrificing accuracy.
Methodology
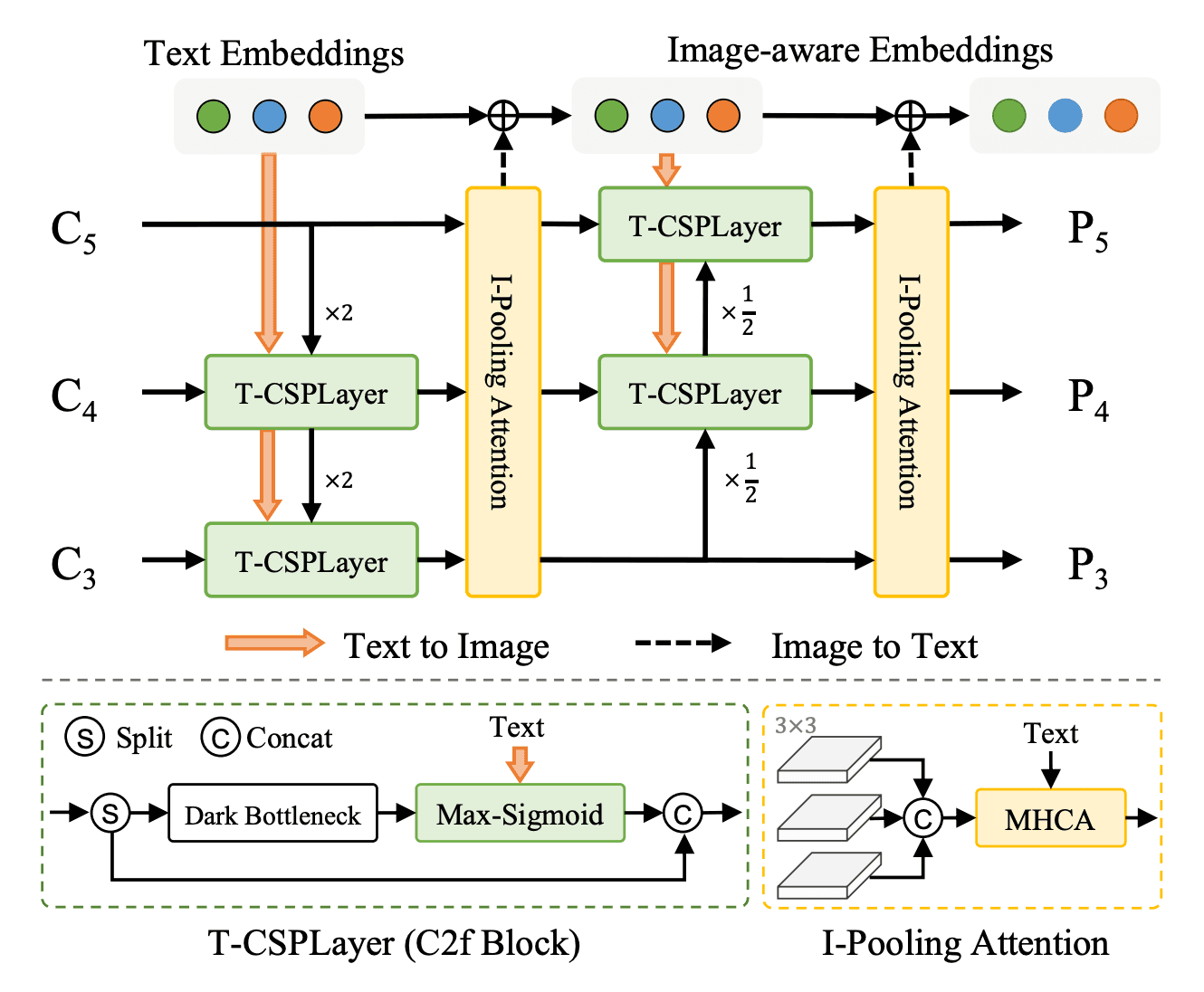
Illustration of the RepVL-PAN. The proposed RepVLPAN adopts the Text-guided CSPLayer (T-CSPLayer) for injecting language information into image features and the Image Pooling Attention (I-Pooling Attention) for enhancing image-aware text embeddings.
YOLO-World introduces a Re-parameterizable Vision-Language Path Aggregation Network (RepVL-PAN) and region-text contrastive loss, enhancing the interaction between visual and linguistic information for wide-ranging object detection in a zero-shot manner.
Significant Results
On the challenging LVIS dataset, YOLO-World not only outperforms many state-of-the-art methods in accuracy and speed but also shows remarkable performance on downstream tasks like object detection and open-vocabulary instance segmentation.
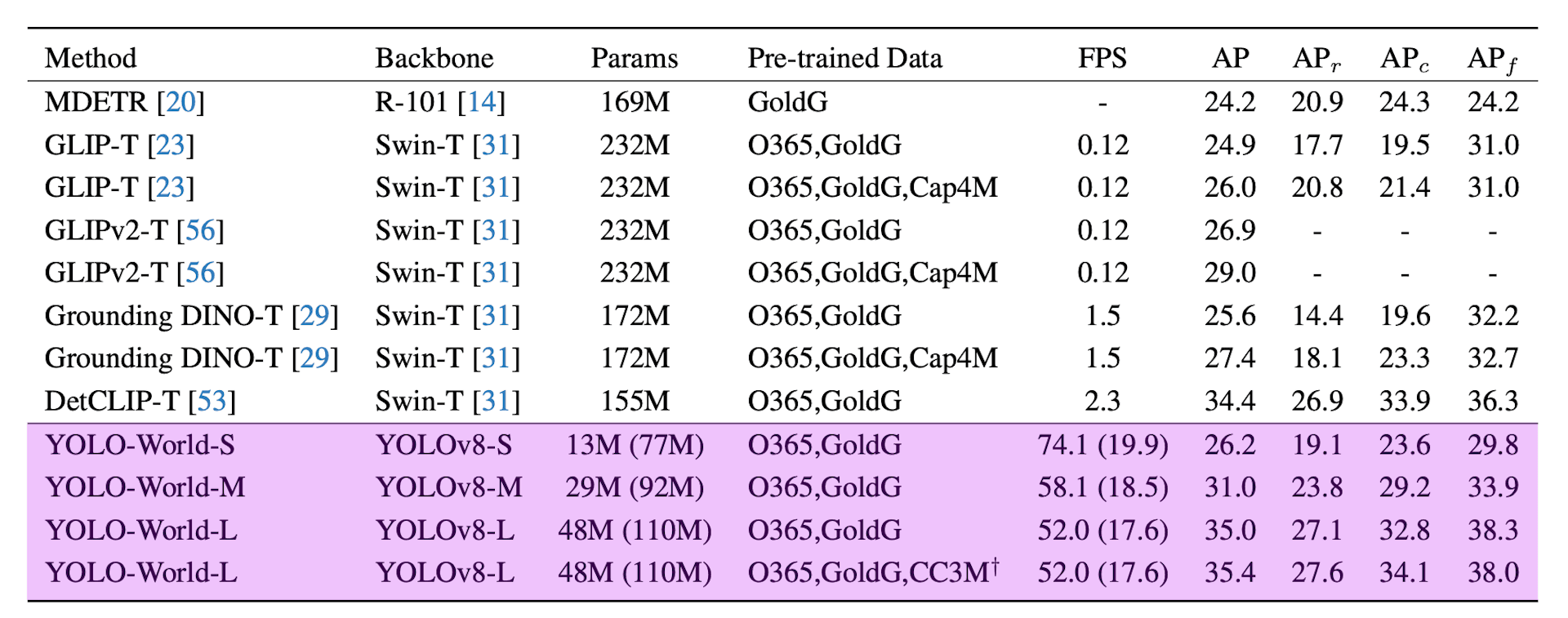
Zero-shot Evaluation on LVIS. Source YOLO-World paper.
Looking Ahead
YOLO-World marks a significant step in making open-vocabulary object detection faster, cheaper, and more accessible, paving the way for innovative applications like open-vocabulary video processing and edge deployment. This model's introduction challenges the status quo, opening new possibilities for real-world applications that were previously impossible due to the limitations of existing detection technologies.
Getting Started with YOLO-World
For those eager to dive into YOLO-World, there's a quick guide to get you started. For more details, visit the YOLO-World Github repository.
There's also a hugging face space demo Hugging-face yolo world demo